Exploring new frontiers in machine learning
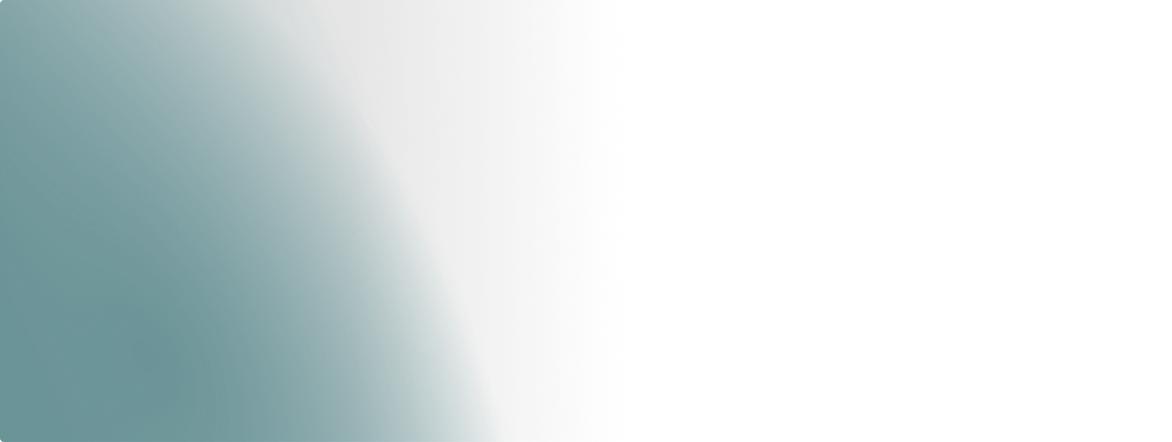
We believe deep learning is the future of algorithmic trading. To get us there, our machine learning team creates the neural network models driving our trading strategies, and builds the infrastructure that make training and inference possible.
What’s unique about machine learning at Jane Street?
We break new ground in predictive modeling, developing approaches that have never been tried before. Here’s some of what we’re up against:
- Financial markets produce a torrent of data, mostly noise.
- We need ultra-low latency systems just to process it.
- Market data is “regime-y,” in that it undergoes frequent structural changes in reaction to things like pandemics, elections, new regulations—even swings in the collective mood.
- Identifying these distinct distributions can be tricky, let alone learning against them.
- And just when we think we’ve got it, our own actions influence the data we’re trying to model (itself a very fun problem to think about).
Thousands
of H100s/200s and growing
$190
billion
daily filled dollars
323 petabytes
current storage
What does machine learning look like at Jane Street?
- No silos. Researchers, engineers, and traders sit a few feet away from each other and work together to train models, build systems, and run trading strategies.
- Depending on the day, we might dive deep into market data, tune hyperparameters, debug distributed training, or study our model’s trades in production.
- We build on the latest papers in LLMs, computer vision, RL, training libraries, cuda kernels, or whatever else we need to train good models.
- We invent our own set of architectures, tricks, and optimizations that work for trading.
Opportunities
Help us pioneer the future of finance.
Open Roles
Welcome Dwarkesh Fans,
We’re Dwarkesh fans too and we’re pleased to be an ongoing sponsor of his content. Learn more about what we are doing in the area of machine learning.
Neural networks are powerful black box models, but at Jane Street, we like to know what’s going on inside. Can you crack our neural net puzzle?